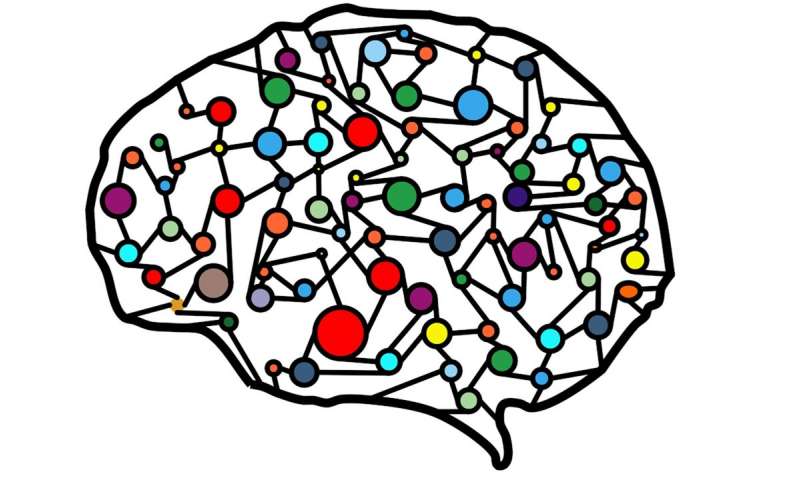
Wait, what? Creating an AI can be way worse for the planet than a car? Think carbon footprint. That is what a group at the University of Massachusetts Amherst did. They set out to assess the energy consumption that is needed to train four large neural networks.
Their paper is currently attracting attention among tech watching sites. It’s titled “Energy and Policy Considerations for Deep Learning in NLP,” by Emma Strubell, Ananya Ganesh and Andrew McCallum.
This, said Karen Hao, artificial intelligence reporter for MIT Technology Review, was a life cycle assessment for training several common large AI models.
“Recent progress in hardware and methodology for training neural networks has ushered in a new generation of large networks trained on abundant data,” said the researchers.
What is your guess? That training an AI model would result in a “heavy” footprint? “Somewhat heavy?” How about “terrible?” The latter was the word chosen by MIT Technology Review on July 6, Thursday, reporting on the findings.
Deep learning involves processing very large amounts of data. (The paper specifically examined the model training process for natural-language processing, the subfield of AI that focuses on teaching machines to handle human language, said Hao.) Donna Lu in New Scientist quoted Strubell, who said, “In order to learn something as complex as language, the models have to be large.” What price making models obtain gains in accuracy? Roping in exceptionally large computational resources to do so is the price, causing substantial energy consumption.
Hao reported their findings, that “the process can emit more than 626,000 pounds of carbon dioxide equivalent—nearly five times the lifetime emissions of the average American car (and that includes manufacture of the car itself).”
These models are costly to train and develop—-costly in the financial sense due to the cost of hardware and electricity or cloud compute time, and costly in the environmental sense. The environmental cost is due to the carbon footprint. The paper sought to bring this issue to the attention of NLP researchers “by quantifying the approximate financial and environmental costs of training a variety of recently successful neural network models for NLP.”
How they tested: To measure environmental impact, they trained four AIs for one day each, and sampled the energy consumption throughout. They calculated the total power required to train each AI by multiplying this by the total training time reported by each model’s developers. A carbon footprint was estimated based on the average carbon emissions used in power production in the US.
What did the authors recommend? They went in the direction of recommendations to reduce costs and “improve equity” in NLP research. Equity? The authors raise the issue.
“Academic researchers need equitable access to computation resources. Recent advances in available compute come at a high price not attainable to all who desire access. Most of the models studied in this paper were developed outside academia; recent improvements in state-of-the-art accuracy are possible thanks to industry access to large-scale compute.”
The authors pointed out that “Limiting this style of research to industry labs hurts the NLP research community in many ways.” Creativity is stifled. Good ideas are not enough if the research team lacks access to large-scale compute.
“Second, it prohibits certain types of research on the basis of access to financial resources. This even more deeply promotes the already problematic ‘rich get richer’ cycle of research funding, where groups that are already successful and thus well-funded tend to receive more funding due to their existing accomplishments.”
The authors said, “Researchers should prioritize computationally efficient hardware and algorithms.” In this vein, the authors recommended an effort by industry and academia to promote research of more computationally efficient algorithms, and hardware requiring less energy.
What’s next? The research will be presented at the Annual Meeting of the Association for Computer Linguistics in Florence, Italy in July.